Advanced and Applied AI on Microsoft Azure
10 Steps to Adopting Artificial Intelligence in Business
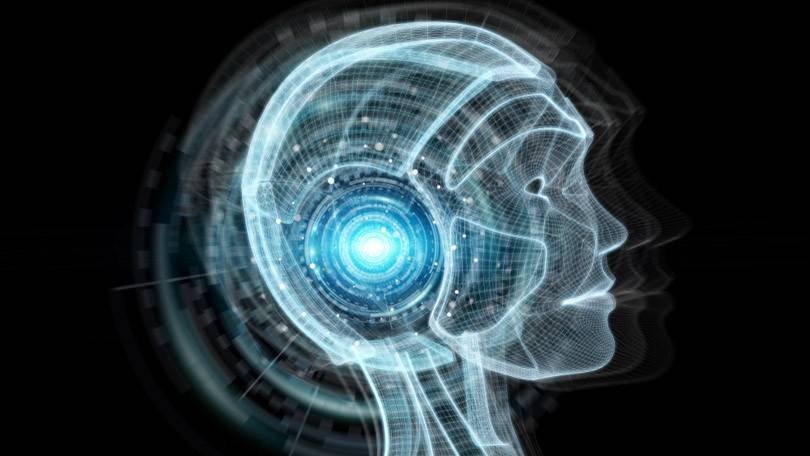
BY ROB MARVIN
What does artificial intelligence (AI) in real-world business scenarios look like? Check out these tips for integrating machine learning, deep learning algorithms, and more into your existing products and services.
Artificial intelligence (AI) is clearly a growing force in the technology industry. AI is taking center stage at conferences and showing potential across a wide variety of industries, including retail and manufacturing. New products are being embedded with virtual assistants, while chatbots are answering customer questions on everything from your online office supplier’s site to your ” class=”aioseop-link”>web hosting service provider’s support page. Meanwhile, companies such as Google, Microsoft, and Salesforce are integrating AI as an intelligence layer across their entire tech stack. Yes, AI is definietely having its moment.
This isn’t the AI that pop culture has conditioned us to expect; it’s not sentient robots or Skynet, or even Tony Stark’s Jarvis assistant. This AI plateau is happening under the surface, making our existing tech smarter and unlocking the power of all the data that enterprises collect. What that means: Widespread advancement in machine learning (ML), computer vision, deep learning, and natural language processing (NLP) have made it easier than ever to bake an AI algorithm layer into your software or cloud platform.
For businesses, practical AI applications can manifest in all sorts of ways depending on your organizational needs and the business intelligence (BI) insights derived from the data you collect. Enterprises can employ AI for everything from mining social data to driving engagement in customer relationship management (CRM) to optimizing logistics and efficiency when it comes to tracking and managing assets.
ML is playing a key role in the development of AI, noted Luke Tang, General Manager of TechCode’s Global AI+ Accelerator program, which incubates AI startups and helps companies incorporate AI on top of their existing products and services.
“Right now, AI is being driven by all the recent progress in ML. There’s no one single breakthrough you can point to, but the business value we can extract from ML now is off the charts,” Tang said. “From the enterprise point of view, what’s happening right now could disrupt some core corporate business processes around coordination and control: scheduling, resource allocation and reporting.” Here we provide tips from some experts to explain the steps businesses can take to integrate AI in your organization and to ensure your implementation is a success.
1. Get Familiar With AI
Take the time to become familiar with what modern AI can do. The TechCode Accelerator offers its startups a wide array of resources through its partnerships with organizations such as Stanford University and corporations in the AI space. You should also take advantage of the wealth of online information and resources available to familiarize yourself with the basic concepts of AI. Tang recommends some of the remote workshops and online courses offered by organizations such as Udacity as easy ways to get started with AI and to increase your knowledge of areas such as ML and predictive analytics within your organization.
The following are a number of online resources (free and paid) that you can use to get started:
- Coursera’s Intro to AI course and UPGRAD’s Artificial Intelligence Program
- Udemy’s online course: Artificial Intelligence & Machine Learning for Business
- edX’s online AI course, offered through Columbia University
- Microsoft’s open-source Cognitive Toolkit (previously known as CNTK) to help developers master deep-learning algorithms
- Deep Learning with Tensorflow, Keras and PyTorch LiveLessons (Video Training), 2nd Edition by Addison-Wesley Professional
- AI Resources, an open-source code directory from the AI Access Foundation
- The Association for the Advancement of Artificial Intelligence (AAAI)’s Resources Page
- MonkeyLearn’s Gentle Guide to Machine Learning
- Stephen Hawking and Elon Musk’s Future of Life Institute
- OpenAI, an open industry and academia-wide deep-learning initiative
2. Identify the Problems You Want AI to Solve
Once you’re up to speed on the basics, the next step for any business is to begin exploring different ideas. Think about how you can add AI capabilities to your existing products and services. More importantly, your company should have in mind specific use cases in which AI could solve business problems or provide demonstrable value.
“When we’re working with a company, we start with an overview of its key tech programs and problems. We want to be able to show it how natural language processing, image recognition, ML, etc. fit into those products, usually with a workshop of some sort with the management of the company,” Tang explained. “The specifics always vary by industry. For example, if the company does video surveillance, it can capture a lot of value by adding ML to that process.”
3. Prioritize Concrete Value
Next, you need to assess the potential business and financial value of the various possible AI implementations you’ve identified. It’s easy to get lost in “pie in the sky” AI discussions, but Tang stressed the importance of tying your initiatives directly to business value.
“To prioritize, look at the dimensions of potential and feasibility and put them into a 2×2 matrix,” Tang said. “This should help you prioritize based on near-term visibility and know what the financial value is for the company. For this step, you usually need ownership and recognition from managers and top-level executives.”

4. Acknowledge the Internal Capability Gap
There’s a stark difference between what you want to accomplish and what you have the organizational ability to actually achieve within a given time frame. Tang said a business should know what it’s capable of and what it’s not from a tech and business process perspective before launching into a full-blown AI implementation.
“Sometimes this can take a long time to do,” Tang said. “Addressing your internal capability gap means identifying what you need to acquire and any processes that need to be internally evolved before you get going. Depending on the business, there may be existing projects or teams that can help do this organically for certain business units.”

5. Bring In Experts and Set Up a Pilot Project
Once your business is ready from an organizational and tech standpoint, then it’s time to start building and integrating. Tang said the most important factors here are to start small, have project goals in mind, and, most importantly, be aware of what you know and what you don’t know about AI. This is where bringing in outside experts or AI consultants can be invaluable.
“You don’t need a lot of time for a first project; usually for a pilot project, 2-3 months is a good range,” Tang said. “You want to bring internal and external people together in a small team, maybe 4-5 people, and that tighter time frame will keep the team focused on straightforward goals. After the pilot is completed, you should be able to decide what the longer-term, more elaborate project will be and whether the value proposition makes sense for your business. It’s also important that expertise from both sides—the people who know about the business and the people who know about AI—is merged on your pilot project team.”

6. Form a Taskforce to Integrate Data
Tang noted that, before implementing ML into your business, you need to clean your data to make it ready to avoid a “garbage in, garbage out” scenario. “Internal corporate data is typically spread out in multiple data silos of different legacy systems, and may even be in the hands of different business groups with different priorities,” Tang said. “Therefore, a very important step toward obtaining high-quality data is to form a cross-[business unit] taskforce, integrate different data sets together, and sort out inconsistencies so that the data is accurate and rich, with all the right dimensions required for ML.”
7. Start Small
Begin applying AI to a small sample of your data rather than taking on too much too soon. “Start simple, use AI incrementally to prove value, collect feedback, and then expand accordingly,” said Aaron Brauser, Vice President of Solutions Management at M*Modal, which offers natural language understanding (NLU) tech for health care organizations as well as an AI platform that integrates with electronic medical records (EMRs).
A specific type of data could be information on certain medical specialties. “Be selective in what the AI will be reading,” said Dr. Gilan El Saadawi, Chief Medical Information Officer (CMIO) at M*Modal. “For example, pick a certain problem you want to solve, focus the AI on it, and give it a specific question to answer and not throw all the data at it.”

8. Include Storage As Part of Your AI Plan
After you ramp up from a small sample of data, you’ll need to consider the storage requirements to implement an AI solution, according to Philip Pokorny, Chief Technical Officer (CTO) at Penguin Computing, a company that offers high-performance computing (HPC), AI, and ML solutions.
“Improving algorithms is important to reaching research results. But without huge volumes of data to help build more accurate models, AI systems cannot improve enough to achieve your computing objectives,” Pokorny wrote in a white paper entitled, “Critical Decisions: A Guide to Building the Complete Artificial Intelligence Solution Without Regrets.” “That’s why inclusion of fast, optimized storage should be considered at the start of AI system design.”
In addition, you should optimize AI storage for data ingest, workflow, and modeling, he suggested. “Taking the time to review your options can have a huge, positive impact to how the system runs once its online,” Pokorny added.
9. Incorporate AI as Part of Your Daily Tasks
With the additional insight and automation provided by AI, workers have a tool to make AI a part of their daily routine rather than something that replaces it, according to Dominic Wellington, Global IT Evangelist at Moogsoft, a provider of AI for IT operations (AIOps). “Some employees may be wary of technology that can affect their job, so introducing the solution as a way to augment their daily tasks is important,” Wellington explained.
He added that companies should be transparent on how the tech works to resolve issues in a workflow. “This gives employees an ‘under the hood’ experience so that they can clearly visualize how AI augments their role rather than eliminating it,” he said.
10. Build With Balance
When you’re building an AI system, it requires a combination of meeting the needs of the tech as well as the research project, Pokorny explained. “The overarching consideration, even before starting to design an AI system, is that you should build the system with balance,” Pokorny said. “This may sound obvious but, too often, AI systems are designed around specific aspects of how the team envisions achieving its research goals, without understanding the requirements and limitations of the hardware and software that would support the research. The result is a less-than-optimal, even dysfunctional, system that fails to achieve the desired goals.”
To achieve this balance, companies need to build in sufficient bandwidth for storage, the graphics processing unit (GPU), and networking. Security is an oft-overlooked component as well. AI by its nature requires access to broad swaths of data to do its job. Make sure that you understand what kinds of data will be involved with the project and that your usual security safeguards — encryption, virtual private networks (VPN), and anti-malware — may not be enough.
“Similarly, you have to balance how the overall budget is spent to achieve research with the need to protect against power failure and other scenarios through redundancies,” Pokorny said. “You may also need to build in flexibility to allow repurposing of hardware as user requirements change.”
==============================================================
BOOKS FROM Pearson FT Press

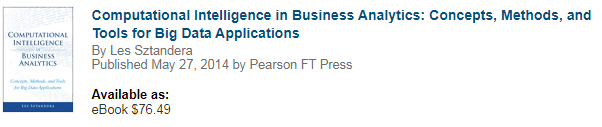